Why Vision-Guided Systems Elevate Assembly Line Precision
Robotic automation provides substantial precision improvements over manual workflows. However, not all robots are created equal. Vision-guided systems can drive these benefits even further for more accurate, efficient and resilient automation.
Advances in machine learning technology have made once-unreliable vision-guided robots an attainable, commercially viable reality. These systems improve assembly line precision in many ways.
Versatility
First and most notably, vision-guided systems are more versatile than their counterparts. Many manufacturers find that what they gain in productivity, they lose in flexibility after implementing robotics. Machine vision helps by providing a wider data set for robots to react to.
Machine vision enables robots to identify components and materials at different angles. Consequently, they can tell if they must move it to work on it, whereas conventional alternatives only operate if parts are in the same position every time. This added flexibility reduces slowdowns or errors from misaligned components and decreases the need for perfection earlier in the assembly line.
Workflow Consolidation
That versatility also lets vision-capable robots consolidate once-separate steps in the workflow. They can move components around to find and work on different areas, even when each one may have slight variations. Consequently, they can accomplish more before sending it further down the line.
Reducing the number of production steps limits room for errors that may affect downstream processes. Because vision-guided machines can accomplish more tasks, they also let manufacturers use fewer robots overall, minimizing equipment costs. With more room on the budget, businesses have more opportunities for optimization and maintenance.
Accident Prevention
Many of vision systems’ most publicized benefits focus on how they can recognize what they’re working with. However, it’s important to note that they can also tell what they’re not. That includes identifying other equipment, nearby workers and foreign objects.
Robots that recognize humans can stop or move before running into them if they get too close. Object and equipment collisions are the second most common cause of injuries in manufacturing, so that’s a hard benefit to overlook. Hazard identification also lets machines respond to any abnormalities in production, whether cleaning parts when necessary or alerting employees of larger issues.
Automated Quality Control
Similarly, vision-guided systems let manufacturers automate quality checks. While essential, these processes often form bottlenecks in production because humans can’t analyze a product as quickly as robots can assemble one. Machine vision removes that bottleneck.
On top of making quality control more efficient, vision-capable robots are more accurate than human employees. Because they compare visual data to the same references every time, they deliver consistent standards. Robots also can’t get distracted, further reducing errors.
Best Practices for Implementing Vision-Guided Robots
Like all other machines, vision-guided robots are a tool, not a complete solution. Manufacturers that want to experience these benefits to their fullest must stick to several best practices.
Ensure Adequate Lighting
Similarly to humans, machine vision can’t see well in suboptimal lighting. Consequently, manufacturers must provide the right amount, direction and color of light for their vision system to reach its full potential.
What kind of lighting a machine needs depends on the specific system. Directional lighting, which mimics sunlight, is the most common method but may create harsh shadows on some surfaces. Diffuse lighting provides more even images but may reduce contrast.
Manufacturers should consult with their robotics and AI partners to determine what light level, direction and wavelengths best fit their needs. Some testing and adjustment may be necessary.
Use Appropriate ML Models
Manufacturers must also select the right kind of machine-learning model to power these applications. It’s similar to how different robots are ideal for varying applications. Delta robots are best for high volumes but have limited reach, and 3D vision models are more versatile but are harder to train.
The key is right-sizing the model to the application at hand. If a robot doesn’t need to recognize objects’ orientation in 3D space, there’s no need to accept the extra complexity of a 3D model.
The same concept applies to the kind of learning the ML model exhibits. Supervised learning is a better fit for simpler machine vision tasks, but reinforcement learning enables more long-term benefits and optimization if manufacturers can afford the extra time.
Practice Good Data Hygiene
As with all AI applications, vision-guided systems require a lot of data to work effectively. Manufacturers must ensure they have enough information to train their ML models before deployment or risk an unreliable vision system.
AI accuracy is also a matter of data quality, not just quantity. While AI is great at analyzing unstructured information, disorganized data sets don’t work in training, so teams must ensure everything is complete, labeled and organized before using it.
Training data is also most effective when it’s relevant. Train vision systems on the kinds of images and objects they’ll analyze in practice as much as possible.
Perform Regular Assessments
Even with high-quality data and a right-sized model, these systems may not deliver optimal results at first. Consequently, ongoing assessments and adjustments are necessary.
Manufacturers should benchmark their performance before implementing vision-guided robots and set goals accordingly. From there, they should periodically test the system according to the same standards to measure how it’s doing.
For every victory and failure, ask why. Tweak the model, the robot or its surrounding workflow and see what changes. These ongoing adaptations will help find the best combination for high-performing vision systems.
Recognize Talent Gaps
Finally, companies must address AI talent gaps. According to one survey, 68% of executives today face a moderate-to-severe AI skills shortage. Manufacturers may be more likely to experience these obstacles, as the industry is relatively new to machine learning.
Manufacturers that don’t have much in-house AI expertise should work with vision system providers who offer more support. Off-the-shelf models are becoming more common, too, presenting another helpful alternative.
Vision-Guided Systems Have Many Advantages
Vision-guided robots provide a crucial edge over more conventional automated systems. Manufacturers that want to maximize their assembly line precision must capitalize on this technology. Doing so requires understanding common obstacles and how to overcome them, but organizations that manage them can achieve impressive results.
Comments (0)
This post does not have any comments. Be the first to leave a comment below.
Featured Product
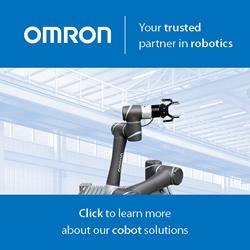