The migration to utilizing AI and ML in mobile systems locally ‘in memory’ has happened very fast within a few short years.
The Invasion of AI/ML into Android Smartphones
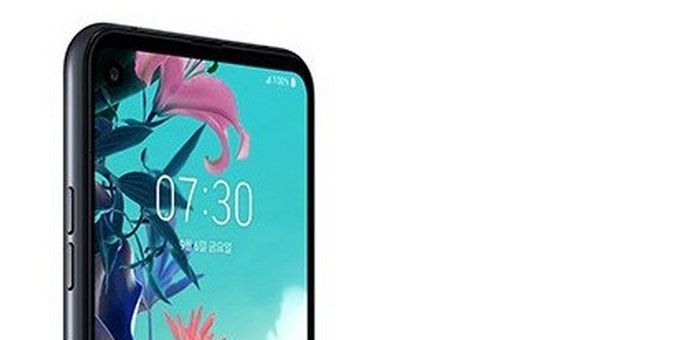
Gerry Purdy | Gyrfalcon Technologies
We’ve been reading about the tremendous developments in AI and ML achieved by Apple the latest iPhone 11 and Tesla in their new neural network chip to help achieve autonomous driving in their cars within the next year or two. Now, Gyrfalcon Technology Inc. (GTI) has developed an AI Neural Accelerator that enables smartphones like the LG Q70 to benefit from high performance & low power all at a much lower price point. We expect to see hundreds of products using GTI AI Accelerator chips before too long.
Artificial Intelligence (AI) and Machine Learning (ML) have been around a long time but are gaining new popularity due to the ability to get these technologies to do some amazing things like beat anyone at chess, recognize someone walking in public from millions of stored faces and other problems which lend themselves to problems that require a lot of parallel processing.
But, up until lately (last couple of years), most AI & ML systems operated in the Cloud that included fast processors that consumed kilowatts of power and large memory arrays. And, a lot of AI mobile systems like Siri and Alexa required the ‘heavy lifting’ to be done in Cloud not on the local device. But, that’s all changing at a very rapid pace.
The old, traditional way of doing AI & ML is shown on the left side in Figure 2. Here, the application that required the use of AI setup the problem locally, such as “What street is this?” (Showing the view of a street). The system would send the question to the Cloud where the solution (big app with big data) would use advanced visual search methods to find the answer and then delver it back to the app. This generally would take significant time (as in seconds not instantaneously), but it all worked.
Then, a number of companies such as NVidia built an integrated AI/ML mobile system with all of the processing and AI parallel memory matrices were built in to the chip. This method quickly branched into two directions:
1. Custom, Closed Systems (Figure 2, upper right) companies like Apple and Tesla decided to build their owwn custom application system integrated circuits (ASIC) that incorporated AI and ML. The best examples have been Apple with the recent introduction of their of the iPhone 11 with the A13 Bionic chip that powers the new video processing and Tesla with their new custom check that is designed to help Tesla cars drive autonomously anywhere in the US.
2. Open, Generic Systems (Figure 2, lower right) companies like Gyrfalcon Tecchnology Inc. have created an open platform with integrated AI and ML. Their best example to date is the recent announcement by LG of their Q70 smartphone that was announced on Monday by GTI (above, Figure 1).
The LG Q70 is in some ways ‘on par’ with the latest smartphones by Apple and Samsung. It has a 6 inch high res display (1080x2310) running Android (version Pie) and a 4000mAh battery. It runs on 5G in Korea. However, it is a breakthrough smartphone in that it has the GTI embedded AI Neural Accelerator chip.
Editors Recommendation "Companies Are Buying Services Instead of Robots"
You can see that these kind of applications cannot easily be done in a standard CPU and memory. The GTI chip (named Lightspeeur®) perform at trillions of operations a second due to
its matrix of 168 x 168 cells that each have memory and a processing unit. The architecture of the GTI AI Neural Accelerator is shown in Figure 3.
It is worth noting that in order to make good use of any/all of these ML systems in memory, developers have to ‘set it up’ in order to gain value. These AI accelerators with neural nets that provide ML functions are basically empty like a baby’s brain at birth: great potential but both have to be trained in order to do insanely great things.
In the case of Apple’s and Tesla’s custom AI/ML chips, they both utilize the inventory of the surroundings to get it to work, e.g. Tesla’s custom AI chip has the experiences of a million vehicles are sensing the roads and environment which ‘trains’ the neural net so that all owners/drivers gain that benefit. Later, the Tesla chip will form the basis of its autonomous driving program it will put that experience to work, e.gg. the Tesla autonomous driving system will, in effect, think “Oh, I know what to do next from the experience of all the other Tesla cars that have been here before.”
In Apple’s case, they use ML in the A13 Bionic chip to constantly learn from how the iPhone is being used and then optimizing the performance by only using power to do things that the user wants to get done. Before, everything got powered as there wasn’t any machine learning process to enable such optimization.
In order to assist developers with the setup process, GTI provides a number of tools to accomplish this task. This is shown in Figure 4.
In the GTI case, the system can be trained do a number of things such as:
- Object detection - You feed in lots of objects and then let the chip easily detect similar objects ‘on the fly’
- Facial recognition You feed in lots of faces withh the specific features and then it will detect those features and people in real time
- Voice ID & Recognition You feed in a lot of voices with known featurres and the system will then recognize them in real time
- Gestures You feed in a lot of gestures with known features and the system will then be able to identify gestures in real time.
This kind of processing is likely to migrate throughout the world of Internet of Things (IoT). According to IDC, they estimate that by 2022, 25% of endpoint devices will execute AI algorithms (inference for neural network applications).
Strategic Insights
The migration to utilizing AI and ML in mobile systems locally ‘in memory’ has happened very fast within a feww short years. It was led by custom efforts where the entire process could be designed and engineered in house: companies like Apple and Tesla.
But the far great part of the IoT industry is now being addressed by companies like GTI which provide very similar capabilities but at much lower cost.
The introduction of the LG Q70 smartphone is just the start. We’ll see hundreds of products with in-memory AI/ML before too long. And, the best part: the user experience is going to improve in ways we can only begin to imagine.
If you like this article you may like "Adopting Service Robot Technology"
The content & opinions in this article are the author’s and do not necessarily represent the views of RoboticsTomorrow
Comments (0)
This post does not have any comments. Be the first to leave a comment below.
Featured Product
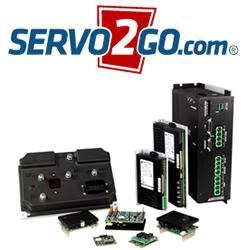