Image Dataset Collection for AI/ML Applications
In the ever-progressing domain of Artificial Intelligence (AI) and Machine Learning (ML), the bedrock of cutting-edge models is closely intertwined with meticulously curated image datasets. This comprehensive article embarks on an investigative journey, probing the intricacies, methodologies, challenges, and transformative impacts associated with the acquisition of image datasets. The exploration delves profoundly into the domain of data that propels the cognitive abilities of machine learning algorithms, uncovering the intricate interplay between these datasets and the evolution of intelligent systems.
In the ever-progressing domain of Artificial Intelligence (AI) and Machine Learning (ML), the bedrock of cutting-edge models is closely intertwined with meticulously curated image datasets. This comprehensive article embarks on an investigative journey, probing the intricacies, methodologies, challenges, and transformative impacts associated with the acquisition of image datasets. The exploration delves profoundly into the domain of data that propels the cognitive abilities of machine learning algorithms, uncovering the intricate interplay between these datasets and the evolution of intelligent systems.
The Essence of Image Datasets in AI/ML:
Before we delve into the nuances of image dataset collection, it's crucial to grasp the indispensable role these datasets play in shaping the capabilities of AI/ML applications. Image datasets serve as the bedrock for training machine learning models, providing the necessary visual input for algorithms to learn, generalize, and make predictions. The quality, diversity, and representativeness of these datasets are paramount in determining the efficacy and accuracy of AI models.
Defining Image Dataset Collection:
Image dataset collection is the meticulous process of gathering, curating, and organising a diverse array of images to form a comprehensive dataset. The objective is to encapsulate a broad spectrum of visual information, allowing machine learning models to generalize effectively across various scenarios.
Importance of Annotated Data:
Annotated data, where images are labeled with relevant information, is a critical aspect of image datasets. Annotations provide context and guidance to machine learning algorithms during the training process, enabling them to understand and categorise visual elements accurately.
Diversity and Representativeness:
A high-quality image dataset must embody diversity in terms of objects, scenes, lighting conditions, and perspectives. Representativeness ensures that the model encounters a wide range of real-world scenarios, enhancing its ability to perform well in diverse applications.
Volume and Scalability:
The size of an image dataset is pivotal for training robust models. A larger dataset allows models to learn from a more extensive range of examples, improving their ability to generalize. Additionally, scalability is essential to accommodate the evolving demands of AI applications as they encounter new data.
Ethical Considerations:
As image datasets play a significant role in shaping AI model behavior, ethical considerations become crucial. Ensuring fairness, avoiding biases, and respecting privacy are essential aspects that need to be addressed in the collection and curation of image datasets.
The Process of Image Dataset Collection:
Gaining insights into the methodologies employed in image dataset collection provides a clearer understanding of the meticulous steps involved in this crucial process.
Domain-Specific Identification:
The process begins with the identification of specific domains relevant to the application. Whether it's autonomous vehicles, healthcare imaging, or facial recognition, each domain necessitates a tailored approach to gathering contextually relevant images.
Annotation and Labeling:
Skilled annotators meticulously label images with the necessary information, including object identification and categorization. The annotation process provides the groundwork for training models accurately and effectively.
Diversity as a Priority:
Recognizing the significance of diverse datasets, the collection process prioritizes inclusivity in terms of ethnicity, gender, and environmental conditions. This approach ensures that the model is trained on a broad spectrum of inputs.
Quality Assurance Measures:
Rigorous quality assurance measures are implemented to eliminate inconsistencies and errors in the datasets. This involves a combination of automated tools and manual checks to guarantee the highest standards of accuracy and reliability.
Scalability for Future Needs:
The datasets are designed to scale, accommodating the growing requirements of AI applications. This scalability ensures that models trained on these datasets can adapt to evolving data demands and stay relevant over time.
Challenges in Image Dataset Collection:
While image dataset collection is pivotal, it is not without its challenges. Several factors contribute to the complexity of this process.
Safeguarding data privacy and security presents a substantial challenge, particularly when handling sensitive images or personal information. The implementation of stringent security protocols and the adherence to ethical practices in handling data become essential in overcoming this challenge.
Bias and Fairness:
Avoiding biases in image datasets is an ongoing challenge. Biassed datasets can lead to discriminatory outcomes in AI applications. Emphasising fairness in dataset collection helps mitigate this challenge.
Annotation Consistency:
Maintaining consistency in annotations across a large dataset is challenging. Rigorous quality control measures are essential to ensuring that annotations are accurate and consistent throughout the dataset.
Volume and Diversity:
Balancing the need for both volume and diversity in datasets poses a challenge. Gaining the right equilibrium is critical for training effective and robust models.
GTS's Expertise in Image Dataset Collection:
As a prominent player in the AI/ML domain, Globose Technology Solutions (GTS) has distinguished itself in the field of image dataset collection. The company's approach combines advanced tools with expert human insight, elevating the process to a level of precision and quality that sets it apart.
Featured Product
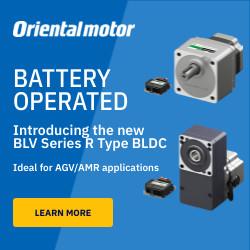