Innovative AI Targets the Problem of Drivable Path Detection in Winter Weather
Driving in extreme winter weather can be dangerous. Ice and snow can make roads treacherous, causing tires to lose their grip, and poor visibility makes it difficult to spot the riskiest patches. This is an issue for even experienced drivers and an even bigger one for self-driving cars.
More than 70% of U.S. roads are in snowy regions where these hazards could arise. As the transportation industry inches closer to the future of fully autonomous vehicles, that poses a problem. Self-driving cars must be able to detect drivable paths in winter weather.
The Problem With Winter Weather Path Detection
Winter weather is an issue for self-driving cars for many of the same reasons it is for humans. While cars don’t have eyes, driverless technologies rely on sensors that need a clear view of their surroundings. Just as ice and snow on a windshield can make it difficult for drivers to see, they can cover cameras and sensors.
Systems like radar and lidar help self-driving vehicles “see” without a clear optical view. However, Tesla, arguably the most popular driverless car company, does not use lidar, relying almost entirely on vision-based navigation. Even with lidar and radar, snow and ice can still interfere with sensors and wireless communication, hindering their efficacy.
Finding drivable paths in winter weather also means understanding various snow conditions. Determining how much snow and ice is on a path can be difficult remotely, making it harder for self-driving cars to navigate.
How AI Is Improving to Meet This Challenge
While winter weather poses a challenge for driverless technologies, some solutions are emerging. Recent advances and new techniques in artificial intelligence (AI) provide a potential path forward. Here’s a look at four of the leading solutions.
Testing in Snowy Areas
One way to improve driverless vehicle AI is remarkably straightforward. Automakers must train these machine learning systems in wintry areas if they want them to learn to navigate through snow. Researchers point out that computers can only react to scenarios they’ve encountered before or are programmed to recognize, so self-driving algorithms need winter experience.
Most driverless car training has happened in sunny, clear environments where machine vision can accurately identify objects. If these technologies are to be safe in the long run, though, they need experience in unsafe conditions. That’s why researchers have started to train them in extremely snowy areas.
Putting rudimentary self-driving systems in snow is hazardous, but it gives these algorithms real-world experience. They can then perform better in these scenarios in the future, having relevant data to inform their decisions.
Sensor Fusion
Another step forward for self-driving AI systems is sensor fusion. In most driverless vehicles, the machine learning algorithms at the wheel react to data from various types of sensors. This helps provide a more cohesive picture of the road, but it can complicate some scenarios.
Different sensors have different applications and strengths. Each one only provides a part of the picture, which can be dangerous in snowy conditions, where no one sensor will have a 100% accurate reading. Instead of deciding on which data to act on, the AI in these vehicles must put all these readings together.
That’s the central idea behind sensor fusion: designing AI to take all sensor data into account. Instead of strictly voting on which one is most accurate at a given time, the system will highlight similarities between all of them. This technique will help AI piece together a cohesive picture from the pieces.
Anti-Ice and Snow Features
Another way to improve AI’s path detection in the winter is to protect the car’s sensors. Lower temperatures can affect electrical components in vehicles, causing them to consume more power and potentially slowing the AI. Similarly, snow and ice can block sensors, limiting data quality.
These issues may have simple physical fixes. Miniature windshield wipers can keep camera lenses clear, giving the AI a clearer view of the road to make better decisions. Similarly, chemical de-icers can prevent frost buildup on sensors to ensure they remain accurate in wintry conditions.
Better insulation can help internal electrical components maintain standard operations. The less this equipment deviates from the norm, the more responsive AI will be, which is crucial in hazardous road conditions.
Teaching AI Winter Driving Techniques
Even when AI recognizes the most drivable path, snowy and icy roads are still hazardous. These machines must also understand how to drive in these conditions, as they require different approaches than clear roads. Consequently, developers must program driving AI to understand winter driving techniques.
AI must understand that it takes longer to brake on icy roads, some brake systems lock, and other winter driving factors. Programming this knowledge into AI can help them recognize when they need to drive differently by braking earlier, pumping the brakes if necessary, and going slow.
The machine learning algorithms in self-driving cars need to apply different techniques to various road conditions. Training them to do so will help keep passengers safe, even with winter weather.
Remaining Obstacles
These innovations could substantially improve AI path detection in winter, but this technology still isn’t perfect. Several roadblocks remain that limit self-driving cars in poor road conditions. One of the most significant of these is that no AI advancements can change the physics of driving on the road.
No matter how skilled a driver or AI is, icy roads are still dangerous, as driving technique can’t overcome physics. For example, car tires on dry roads may achieve a 1.0 friction coefficient, but that can drop to 0.08 with ice. Without specialized tires, that little friction poses a considerable risk, regardless of how well an AI can recognize it.
That raises an ethical question with driving AI, too. If AI in self-driving cars can detect that road conditions are too dangerous to drive, should they refuse to do so? Should these AI systems be able to tell their owners it’s too risky to drive, or should they always follow orders? If the former, at what point should they say driving is too unsafe?
These questions become more muddled when considering the safety of other people on the road, too. While these considerations may not be relevant until driving AI becomes more advanced, they deserve attention.
Driving AI Isn’t Perfect, but It Is Improving
Today’s AI is a long way from being able to navigate through harsh winter weather safely. However, while several obstacles remain, recent innovations show promise. As this technology advances, self-driving cars could make winter travel safe, or at least safer than it is now.
Comments (0)
This post does not have any comments. Be the first to leave a comment below.
Featured Product
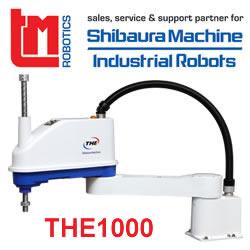