This method teaches a robot how to perform a single example task, while simulation addresses the problem in a more statistical way, conducting tens of thousands of virtual experiments to find the right solution for performing that task.
Teaching Robots to Help People in their Homes
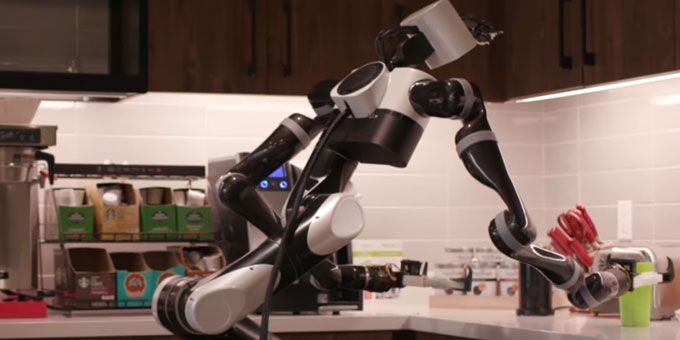
Dan Helmick | Toyota Research Institute
What challenges are inhibiting the feasibility of in-home assistance robots?
The biggest challenge we are facing today is getting robots to effectively operate in a given home. Unlike the structured environment of a factory, where robots are most commonly used today, homes are “the wild west” in terms of their customization and fluidity. Each home is different -- different furniture, different floor plans, different arrangements of things -- and there are constant changes with humans and pets living inside and moving about. Engineering a robot to be able to robustly perform tasks across that extreme variety is a huge technological hurdle that has to be overcome.
How does your teaching approach enable overcoming these technological hurdles?
It is the first step toward fleet learning. Today, we are working on being able to efficiently teach a single robot to do a single task in a in a single home. While achieving that is useful in and of itself, the real impact comes from when we are able to transfer that individual teaching on one robot to a fleet of robots, each in a different location with different operating environments, such that they all can perform the task in their situation. Fleet learning is key to the Cambrian explosion of robotics, the combination of cloud robotics and deep learning, that TRI CEO Dr. Gill Pratt professed in 2015 will lead to an exponential increase in robotic capabilities.
How is the teaching method different than or a complement to the simulation approach that TRI is also pursuing?
This method of one-shot teaching is quite different than the simulation approach that TRI is also pursuing. This method teaches a robot how to perform a single example task, while simulation addresses the problem in a more statistical way, conducting tens of thousands of virtual experiments to find the right solution for performing that task. These differing approaches to solving the problem are at opposite ends of the spectrum. However, in the future, we envision these two methods converging and becoming complementary, where you might perform the actual teaching in simulation and potentially model a wide variety of scenarios and tasks in simulation, and then apply that to teaching robots in an analogous way that we are physically doing it now.
Since this is a first step to achieving your broader vision of Fleet Learning, what must happen next?
Right now, we are teaching execution of a specific task on specific objects in a specific house which can adjust for small variations that each house may have, such as lighting changes or objects in different positions or locations, like a bottle moved to a different shelf of the refrigerator. We are working to simplify this complicated teaching procedure. Thinking further ahead, we want to make a single taught task more generalizable across different objects and different scenarios, so that each taught task can then be used in a more general way.
How do you think others in the robotics community will benefit from the teaching approach?
Our approach is conceptually very simple. Teaching a robot how to perform a task using the robot’s sensory data through an immersive telepresence system can be applied to a wide range of robotics applications. We have been able to make this approach very robust, which can be a pretty powerful concept for the robotics community.
Why is Toyota/TRI investing so many resources into robots?
The rate in growth of the world’s aging society will have global crisis implications. While public policy plays a key role in addressing the socioeconomic impact of the demographic shift, we believe it is critical for robotic capabilities to become more advanced. Developing robots to become household assistants will be critical to enabling people to age in place longer and live a higher quality life. The Toyota Research Institute (TRI) is focused on helping Toyota create and prove the technological breakthroughs necessary to make assistive home robots feasible. Advances in cloud robotics and deep learning is making fleet learning possible, and we are on the cusp of achieving an exponential increase in robotic capabilities toward fulfilling our longer-term goal.
Are there any other projects in the works that you would like to talk about here?
As mentioned, we are really focused on generalization in our pursuit of fleet learning. Our strategy follows three different axes. The first is generalization across different objects. As I mentioned, we currently teach on a specific object and want to transfer that learning to apply to other objects. Second, we are pushing to generalize across scenarios. Right now, we are teaching a robot in a specific home how to perform a task. Our goal is to perform the teaching exercise in a different location and have it apply in that specific home and other homes. For example, we are experimenting with a mock home environment and teaching a task on a particular model refrigerator for a robot to execute that task in somebody's home. The third axis is generalization across robots. Currently, we teach on a on a particular type of robot that is in our fleet, and we always execute that task on that type of robot. But, there are other robots in our fleet, like Toyota’s HSR, and new ones coming down the pipeline, that we want to use. While we are teaching on one particular robot, our plan is to become agnostic as to the robot that is used to perform the task. We have been conducting some initial successful experiments where we teach on one robot, and then execute on a completely different robot. Advancements on each of these three axes of generalization is necessary for us to achieve our broader vision of Fleet Learning for assisting and empowering people in their home.
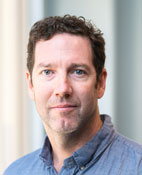
The content & opinions in this article are the author’s and do not necessarily represent the views of RoboticsTomorrow
Comments (0)
This post does not have any comments. Be the first to leave a comment below.
Featured Product
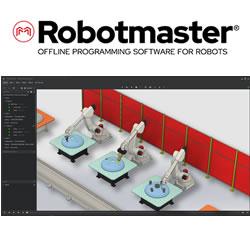