This thought experiment shows why robot localization, the task of maintaining a location position as robots move around, and obstacle avoidance, is so critical to the success of all non-stationary robots.
Indoor Tracking Technologies in Robots & Drones
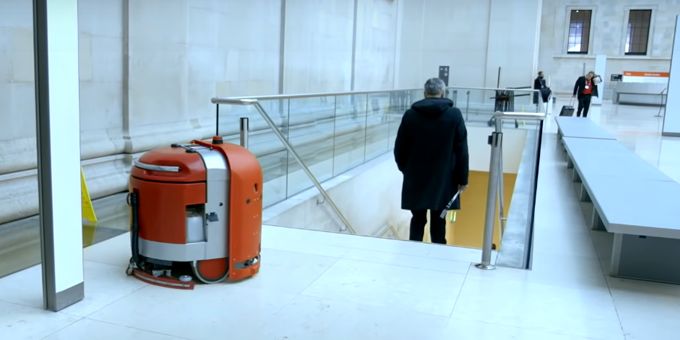
Bruce Krulwich | Grizzly Analytics
Imagine trying to clean the floor in a big site, or carry food to tables in a restaurant, or deliver items between people in a site, or any of the other myriad of tasks that robots are doing, with a blindfold on. That’s right, no sight. Impossible, right? This thought experiment shows why robot localization, the task of maintaining a location position as robots move around, and obstacle avoidance, is so critical to the success of all non-stationary robots.
Tracking location indoors has gotten a lot of attention recently in the world of smartphones and mobile devices. Google Maps has been offering location on indoor maps for years, using cellular and Wi-Fi signals to multilaterate a location estimate. Apple just announced a new chip in their upcoming iPhones for ultra-wideband (UWB) radio, used for more precise distance and location measurements. And over two hundred technology vendors are offering a variety of indoor location monitoring, based on many different technologies and used for a wide range of applications. From all this attention, many would think that location tracking is a solved problem.
Robots, however, have several unique requirements and challenges beyond those of mobile devices, and different technologies are needed in order to keep the robots location-aware. And while we discuss robots, keep in mind that everything applies to autonomous vehicles, drones and other devices in motion.
The first challenge that robots face is that their wheels slip.
Many robots track their locations, relative to a known starting point, by precisely measuring the movement of all of their wheels. Basic geometry tells us that every full rotation of a wheel moves that point on the robot forward by 2πr, or a distance of double π multiplied by the radius of the wheel. Combining measurements of each wheel with details of where each wheel is on the robot, based on the geometry of how the wheels turn around each other on curves, can, in principle, allow precise tracking of a robot’s location as it moves around a site. Robots on wheels can actually do this much more effectively than motion sensing on smartphones, where error creeps in based on how the phone is being held and how the phone user is walking or running around a site. But even with the accuracy of robot wheel sensors, if the wheels slip, which is very likely for a floor-cleaner robot, or if the robot is bumped, as can happen in real-world robot settings, then the robot’s location will change in a way that the sensors cannot measure, and over time the robot’s measurement of its own location will get worse and worse.
The second challenge is that robots need more accuracy than mobile applications. A lot more.
If you want your floor cleaning robot to clean the corners of hallways and the edges along furniture, it will not be able to do a good job if its location estimate is two meters off from its real location. If you want your restaurant’s robot waiter to move between the tables without bumping them, a meter or so of inaccuracy will leave the robot frozen in place lacking a safe route between tables. For these and many other robot tasks, location accuracy needs to be in the millimeters or centimeters, not meters.
The third challenge is a dynamic environment. Robots need to not only move around an area, but also to avoid things that are moving in their way.
A robot that can move effectively around a warehouse, factory floor, store or restaurant, when it has a map and knows where everything is, may not be able to do so when there are people walking around the site at the same time or when objects at the site are moved from one place to another. Knowing the robot’s location and the location of things around the site is not enough, the robot needs to be able to sense its environment dynamically and react accordingly. So classic approaches used in mobile applications, in which a smartphone user navigates using a blue dot on an indoor map, will not be sufficient for a robot that needs to know what else is on the map.
Editors Recommendation "Artificial Intelligence Drives Advances in Collaborative Mobile Robots"
So how are robots on the market tracking their locations?
CleanFix, maker of commercial cleaning machines and robots based in Switzerland, uses a combination of “dead reckoning” motion sensing and radar-like laser measurements. Their robots have an attached laser component that measures the distance between the robot and the nearest walls or objects, over a 270 degree range of directions, soon to be 360 degrees on future models. These laser measurements enable the robot to take a rough location estimate from dead reckoning and refine it to be accurate within 2-3 millimeters.
Keenon Robotics, maker of robot waiters for restaurants and other highly specialized robots, uses visual “tags” that are mounted on the ceiling. Each tag is 1-2 square centimeters in size and is seen by upward-facing cameras on the robots. The robots can calculate their positions, based on seeing the tags, to within 1cm. The visual tags have the advantage of being very easy to install and configure, generally requiring 1-2 hours to put up the tags and a few hours for the robot to self-learn how to move around the site based on the tags.
The challenge that these and other robot makers are facing is the tradeoff between accuracy/performance and price. Laser-based radar components are expensive and add size and complexity to a robot. For a high-end commercial robot like CleanFix this may be reasonable, but makers of lower-priced robots are often interested in lower-price options. This is where newer technologies from the mobile realm fit in.
Accuware, based in the USA, are the makers of visual technology that can learn autonomously to locate a robot or other device as it moves around a site. Their SLAM (simultaneous localization and mapping) technology does this based on a video stream from a standard front-facing camera. In essence, their system tracks the robot’s or device’s location the same way that people do, by looking at the scene around them and measuring their own movement compared to things they see. At the same time, their system learns about the environment based on what they see, and can localize themselves more accurately the next time they see the same scene.
For robots and devices with a single camera, Accuware’s technology achieves centimeter level accuracy. But when a robot has 2 cameras, similar to a human being’s 2 eyes, their technology is more accurate and reliable, using stereoscopic sight to measure the distance to things that they see and to more accurately measure robot rotation.
Visual technologies such as Accuware’s cannot yet achieve the millimeter-level accuracy that lasers can achieve, but they are much less expensive, and can be more resilient to changes in the environment like people walking around, that change the distances between the robot and its surroundings but can be visually distinguished from walls and equipment.
Most importantly, cameras are already installed on many robots, either to enable human remote control or for accountability after-the-fact. Connecting existing cameras wirelessly to a localization system is much easier and cheaper than installing new components or infrastructure.
Other technologies used for robot localization include ultra-wideband (UWB) radio, recently in the limelight because of Apple’s announcement. Several companies are using UWB for robot localization, including iRobot, who according to FCC documentation is using UWB in their Terra robotic lawn mower. UWB can achieve centimeter level accuracy, but requires either that locator devices be installed around the site or that many multiple devices connect in a mesh network.
Because of the challenges in robot localization and the benefits and tradeoffs of the different technologies, robots on the market increasingly use a variety of technologies, often taking what is called a “belt and suspenders” approach of using multiple technologies that complement each other. This is particularly important for robots at lower prices, where it may be impractical to use high-priced laser components.
As the markets for robots, autonomous vehicles and drones continue to grow and spread into new uses, the need for robots to track their own locations accurately and effectively will only go up. Keep an eye on these technologies to enable robots to know where they are.
If you like this article you may like "Smart Gripper for Small Collaborative Robots"
The content & opinions in this article are the author’s and do not necessarily represent the views of RoboticsTomorrow
Comments (0)
This post does not have any comments. Be the first to leave a comment below.
Featured Product
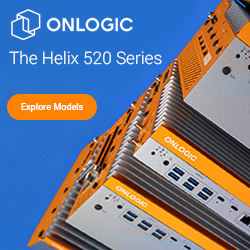