Our goal is to simplify AI so organizations can start deploying new technologies to save time and money. What we offer is a platform of AI and vision-based inspection and traceability apps that are easily customized
Utilizing AI in the Manufacturing Space
Q&A with Ed Goffin | Pleora
Tell us about yourself and your role with Pleora.
I’ve been in the technology industry for about 25 years, working in markets from semiconductors and telecom to AI solutions for manufacturing. I’m responsible for Pleora’s product and marketing strategies for our video connectivity portfolio and expansion into AI for manufacturing.
How is Pleora bringing AI to the manufacturing space?
Machine vision is the backbone for many AI applications in the manufacturing market. Pleora has been in business for over two decades and helped champion the development of machine vision connectivity standards, which are so crucial today, as AI moves onto the manufacturing floor. We also have a wealth of experience in real-time connectivity and processing requirements for mission-critical applications. For example, if you’ve had an X-ray, our solution most likely transmitted images from the panel to processing.
One key trend that helped drive adoption of vision across multiple markets is ease-of-use. Complex camera, sensor, and processing technologies evolved into plug-and-play solutions. We’re bringing that same approach to AI for manufacturing. Our goal is to simplify AI so organizations can start deploying new technologies to save time and money. What we offer is a platform of AI and vision-based inspection and traceability apps that are easily customized for unique workflows to make manufacturing decisions consistent, reliable, and trackable.
What are some of the common misconceptions around AI for manufacturing?
One of the biggest misconceptions is that it’s complex. This was true a few years ago, but more recently there’s been a significant emphasis on making AI tools simpler and easier to use. Our position is that you don’t need to be an expert to develop your own AI algorithm or digitization workflow. With user-friendly drag-and-drop development tools, and customizable app-based templates for common requirements, anyone can develop their own AI-based workflow. This is a huge advantage for a manufacturer aiming to avoid concerns around vendor lock-in and recurring integration and development costs.
What types of problems can AI solve?
Machine vision excels at pass/fail decisions, but it’s difficult to manually program acceptable tolerances. In comparison, AI can be more easily trained to learn around those variable decisions. The example I use is hardwood inspection. It’s very difficult to manually program machine vision to discern between natural grain and a scratch. In comparison, it’s much easier to train AI based on several good and bad images so it can recognize the difference. Basically, thanks to its learning ability AI can help make subjective decisions.
There’s also a significant opportunity for AI in inspection processes that rely on a human decision. AI can help us make the right call on those subjective quality decisions or catch errors as our attention begins to flag over a shift. We’re working with an auto parts manufacturer that relies on human inspection but is adding AI-assistance to catch defects that may be missed or decide if a defect is within operating tolerance.
AI-based visual inspection helps an auto parts manufacturer identify errors and decide if a defect in within an acceptable performance tolerance.
How are manufacturers using AI today?
One key area where manufacturers are deploying AI is around human decision support. While there has been a lot of investment in automation for manufacturing, about 70 percent of processes in the United States still require a human decision. This is especially true for smaller run, customized or seasonal production where it is too expensive and complex to invest in full scale automation.
Visual inspection is one area where AI can help humans consistently make the right decision. A visual inspection app as part of a camera-based system highlights product differences or defects for an operator. This is also one area where, back to the previous question around misconceptions, we can use those initial operator decisions around those defects to train an AI model. As an operator accepts or rejects those initial differences, behind the scenes they are transparently training an AI model. After a few inspections the AI model will begin suggesting the decision to the operator.
While the inspector is catching errors before they reach market, the manufacturer is also gathering a complete record of that inspection including product images and operator notes to provide full traceability on the manual process. For example, we work with an electronics manufacturer that assembles parts for high reliability applications, and having a complete end-to-end record of inspection steps and operator decisions is critical for traceability.
Dica Electronics uses visual inspection as a “second set of eyes” to catch potential production errors while also capturing a full record of product images and operator notes to ensure traceability.
Do you have a recommendation for how manufacturers can deploy AI?
There’s a lot of hype around AI, and too often manufacturers build expectations based on the perfect use case. Quickly, they run into issues. Their application is maybe not quite as straightforward as that perfect use case. A lot of customization is required. A common issue is simply acquiring the images required to build and train an AI model – especially if you’re manufacturing unique and lower volume products.
Often our recommendation is to digitize processes first and scale towards automation. Visual inspection is a good starting point, where you first use machine vision to detect errors and then add AI-based decision support to scale that consistent decision making over a shift or across different workstations. As you digitize that first error-prone process, you’re capturing data that helps guide your next automation decision. Often that’s adding traceability to those visual inspection decisions or incorporating guided work or assembly instructions into the inspection process.
Overall, choose an error-prone process and see how you can use digitization and AI to save you time and money. We’re working with many manufacturers who started with a pilot project around that first troublesome defect or process, gained comfort with the technology, and are now scaling across different workstations or production lines.
What are the biggest hurdles to using AI for manufacturing?
This is a big one and often overlooked; don’t forget about the human in your process.
Even with automation, many processes still require a human decision at some point. This may be as simple as explaining to an operator why you want to automate a process and provide the necessary training so they can apply their expertise in new ways. In a robotic welding application, for example, the goal is to remove the human from the repetitive, dirty, and dangerous work, but still rely on their expert insight and years of training to monitor the process and assess results. Without proper communication and training, humans will be quick to dismiss technology and resist change. That’s just our nature.
Look down the road a few years, where do you see AI for manufacturing and how is Pleora involved in taking it there?
Not too long ago there was a lot of general fear around AI. That general concern, for the most part, is disappearing. That’s thanks to AI technology becoming easier to use and more commonplace in our day-to-day lives. I am amazed at how many decisions I now just leave to the virtual assistant on my smartphone.
We’re at the same point in manufacturing. AI was expensive and complex just a few years ago and primarily restricted to advanced labs, but development tools now make it easier for a quality manager to design and deploy their own AI-assisted workflows. There’s also more focus on how AI technologies can help the human workforce, by removing them from the dull, dirty, and dangerous tasks and assisting their decision-making.
The key for widescale adoption in the manufacturing markets is making these technologies easier for the end-user. That’s our prime focus; giving quality managers a customizable, easy-to-deploy solution so they can reduce manufacturing errors and costs.
The content & opinions in this article are the author’s and do not necessarily represent the views of RoboticsTomorrow
Comments (0)
This post does not have any comments. Be the first to leave a comment below.
Featured Product
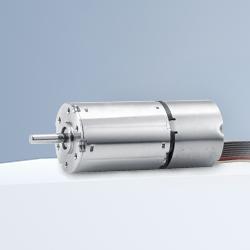