The industry is evolving rapidly in every sector, not just robotics – that’s exactly why legacy architectures don’t make sense for edge AIML going forward
Embracing a Purpose Built AIML System in Robotics
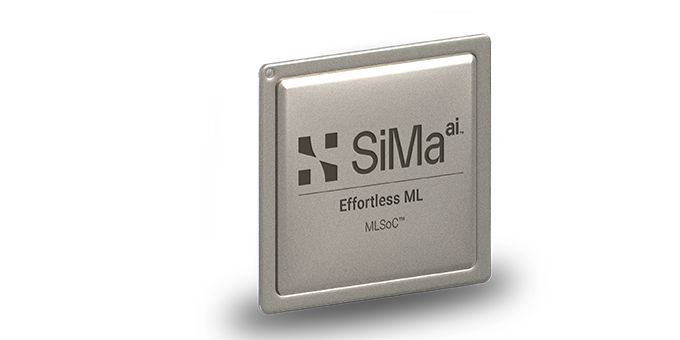
Q&A with Alicja Kwasniewska, Senior Principal ML Architect | SiMa.ai
Tell us about yourself and your role with SiMa.
My background is in AI and data science, specifically deep learning – I’m currently a Senior Principal ML Architect at SiMa.ai, focused on reshaping field operations and unlocking new levels of efficiency, security, and real-time decision making across various industries. I’m also deeply involved in research – last year I was selected as top 10 women in AI for my achievements and contributions to AI research. So far, I’ve published over 30 whitepapers in machine and deep learning topics. I’m actively exploring the challenges and opportunities associated with deploying large multimodal models at the edge.
Prior to joining SiMa.ai, I dedicated five years at Intel developing AI solutions at various Intel AI edge accelerators, as well as in the cloud. I actively contributed to various open source projects such as Intel Swan, Snap or the OpenStack Kolla where I was acting as the Core Reviewer.. I’m particularly interested in AI for image, text, voice, and other data analytics, and focused my PhD research on biomedical engineering and AI for medicine.
SiMa.ai is known for its purpose-built AI/ML edge system solutions. Can you explain how your technology is advancing the capabilities of robots, and how this impacts those who operate them?
On a recent episode of our “OnEdge” educational YouTube series we showcased how SiMa.ai is advancing the capabilities of robots operating at the edge. When it comes to industrial robots, our technology addresses the challenge of deploying machine learning models at the embedded edge. SiMa.ai’s SDK allows developers and companies to seamlessly integrate machine learning models from various frameworks without the need for conversion or optimization outside the toolchain. This flexibility enables the deployment of diverse models developed by separate teams, each using different frameworks or model architectures.
On top of that, we can accelerate the entire pipeline, not only the model itself. Usually the performance bottleneck comes from other portions of your applications, and even though your model achieves good performance, the end to end solution doesn’t work in real time. With SiMa.ai heterogeneous MLSoC and efficient runtime, you achieve real-time processing at the lowest power from the sensor to the output, which is crucial for markets like robotics to ensure smooth operations and fast responses as well as meeting size, weight, and power (SWaP) requirements.
SiMa.ai’s software is designed to be future-proof, accommodating improvements and updates to machine learning models over time. The selection of best quantization schemes and techniques of different models, necessary for accurate but efficient inference on resource-constrained devices, is supported by SiMa.ai’s solution. Our ultimate goal is a simplified, push-button methodology to compile and deploy these models to SiMa.ai’s hardware. This software capabilities combined with memory hierarchy and efficient static scheduling provides a mechanism to quickly port latest model architectures keeping up with the fast pace of AI advances and rapidly update the models to achieve the best AI performance in real-world scenarios, such as manufacturing lines, robotic surgeons, and other robotic platforms.
As a result, operators get the best AI solutions in minutes, without the hassle of updating systems over a prolonged period of time and can get the best support for their tasks and as much automation as needed.
The robotics industry is rapidly evolving. How does SiMa.ai adapt to stay at the forefront of these advancements?
The industry is evolving rapidly in every sector, not just robotics – that’s exactly why legacy architectures don’t make sense for edge AIML going forward. The key is to provide a software-hardware co-designed platform that is purpose-built for AI at the edge. We are a software company, building our own silicon, because we understand the need of the software first approach in the ML world.mFlexibility and ease-of-use are core tenets of our product suite. This differentiation, along with forward-thinking R&D and our agile and automated development methodologies, makes it easy for us to quickly respond to changes in technology and market demands for our customers.
Manufacturing is a great example of this – as factory robots become increasingly popular, we’ve used depth cameras with our Palette software’s model SDK to showcase the practical application of our technology in testing accuracy and performance of different machine learning models, before they ever go into production. Our Palette Edgematic no-code development platform allows for the same but instead of using command line interfaces (CLIs) and APIs, you can use a visual no-code canvas to build a pipeline from pre-optimized blocks with a drag and drop approach. Then, using the integrated performance estimator, you can quickly evaluate pipeline performance and find bottlenecks that could be further optimized across CPU, DSP and ML Accelerator.
SiMa.ai's mission is to make ML at the embedded edge effortless. What does the robotics field have to gain from embracing a purpose built AIML system like SiMa’s – what can they do with SiMa that was previously not possible?
Embracing a purpose-built AIML solution such as SiMa.ai’s offers several advantages for the robotics field:
- Our offering allows users to ingest models from any framework using our SDK, which eliminates the need for pre-processing or conversion of models outside the toolchain. This streamlines the deployment process and allows for the direct loading of models from the training pipeline.
- We also provide future-proof software – as developers seek improvements in models, the software can seamlessly accommodate updates, keeping it aligned with the latest research and topologies. The quantization of models, along with the capability to evaluate accuracy, enables the deployment of high-performance models without compromising functionality.
- Our heterogeneous platform allows for running the entire application on a single chip, not only the ML portion of it. That gives you threefold benefits: 1) you can get the best performance and the lowest power 2) you can simplify your software stack by moving some of the functionality to our chip that was previously deployed on another engine 3) because of that you can also reduce the cost of the entire system.
Overall, SiMa.ai’s AIML solution empowers the robotics industry to deploy, update, and optimize machine learning models efficiently at the embedded edge, opening up opportunities for real-world applications that were previously challenging or not feasible.
Robotics in sectors like healthcare, manufacturing, and logistics are becoming increasingly autonomous. How does SiMa.ai's technology support the development of more intelligent and capable robots in these domains?
In order for something to be truly autonomous, it must have the intelligence to quickly react and adapt, and the performance and efficiency to do so without an exorbitant amount of energy. It’s clear that innovation for software and hardware systems has stagnated in previous years, as the technology to effectively power robotic platforms, healthcare applications, automotive devices, and other machines hasn’t approached what today’s devices require.
SiMa.ai was founded to combat this exact issue. Our Palette software offers a low code, integrated environment for edge ML application development, where customers can effortlessly create, build and profile their solutions. In the past, silicon makers have created one-size-fits-all chips that force innovators to hand-code their programs to match the chips they are buying. We eliminate this learning curve with Palette, so compiling and evaluating any ML model, from framework to silicon, can be done in minutes.
Our chip optimizes software and hardware to save energy without sacrificing performance in the low-powered devices, including robots utilizing the edge. We developed our Machine Learning System-on-Chip (MLSoC) to be compatible with multiple open source and existing legacy applications, so our customers don’t need to write new applications or learn new coding languages to support machine learning.
These are the capabilities required to take today’s devices to the next level with AIML. A single SiMa.ai MLSoC chip can provide 60 frames-per-second real-time processing of complicated pipelines based on multiple neural networks and computer vision algorithms with less than 15 watts of power consumption, delivering a superior performance for an entire customer application. When using our technology in the field, one customer saw a nearly 15X improvement over competitors when using our chip with their computer vision technology.
We’re excited for our customers to do even more with our technology, as we are marching towards enablement of Large Multimodal Models on our platform. With such solutions, robots’ actions can be adjusted based on real-time egocentric observations, as well as past memories to self-adapt to changing environments in which they operate. That will allow for more natural, dynamic, and autonomous actions and continuous improvement, not possible at the embedded edge before.
Ethical considerations and responsible AI are important in the development and deployment of robotics. Can you share SiMa.ai's perspective on ethical AI in robotics, and the steps your company takes to adhere to those standards?
Ensuring that the behavior of artificial intelligence is predictable is a must when implementing the technology into robotics, as failure to do so could cause harm to the workers around these machines, not to mention supply chain breakdowns and product deficiencies. The only way to enable broad adoption and usability of AI is to ensure its creators and users know what to expect from it and can map inference accuracy to what has been achieved during model training and evaluation. This can be challenging when also optimizing for performance and power consumption, but SiMa.ai has been able to match our Palette SDK and various optimization and analytics tools we offer to make it possible at the embedded edge.
Additionally, operating in the embedded edge allows engineers to access unfiltered raw data from various sources quickly, and therefore operate more safely. AIML models on the cloud are constrained to the platform’s bandwidth limitations, and therefore usually operate on non real-time, pre-processed data. This can lead to the omission of important details and patterns that result in inaccurate AIML systems. I believe the embedded edge is the only way to make a real use of multimodal solutions with detailed, accurate and comprehensive analytics.
At the same time, collaborative learning is also very important in many robotics use cases. Humans should be involved in design, evaluation and analysis of the ML solution at the appropriate level to ensure the model behavior is correct. SiMa.ai Palette software allows for full transparency of model optimizations and a set of tools for evaluation and profiling, so that users get full insights into the flow of their optimized applications and models.
What are some challenges that robotics engineers or ML developers face when trying to build ML applications for their embedded edge production solutions, and how does SiMa.ai’s technology resolve those challenges?
Today’s engineers are burdened with the need to embed artificial intelligence into their products and services, but developing that is easier said than done. Many companies don’t have the resources to train a specialist to add AIML to their devices, or their engineers don’t have the time to spend months evaluating and deploying ML applications.
To solve this problem, we developed Palette Edgematic, a no-code approach to creating, evaluating and fine-tuning ML applications from anywhere in the world via a browser. Palette Edgematic enables a “drag and drop,” code-free approach where engineers can create, build and deploy their own models and complete computer vision pipelines automatically in minutes versus months, while evaluating the performance and power consumption needs of their edge ML application in real time. Even for experienced teams who know the ins and outs of ML development, Edgematic allows them to quickly prototype and evaluate their models.
What trends do you see shaping the future of robotics and AI integration?
In the coming years, we’re going to see an increase in solutions that prioritize safety and accuracy in the technology, especially as we see more robotic solutions on the factory floor. We’re already seeing this with advancements in human-to-robot motion retargeting, which will allow engineers to train robots how to perform by describing or physically demonstrating desired actions. As the low-latency required for this AI technology is not possible to run on the cloud, we’ll see an increase in adoption of edge solutions, especially in smart manufacturing ecosystems, where AI-driven systems are seamlessly integrated into every stage of the production process.
AI systems will also start to become more autonomous, especially as companies migrate from the edge to the cloud. As I mentioned earlier, we will see more and more systems utilizing a combination of past memories with current observations obtained by interacting with environments to adjust their behavior to specific domains and scale to new tasks. I believe SiMa.ai’s MLSoC platform and Palette software will drive such innovations at the embedded edge to offer the quickest deployment and real time execution of cutting-edge AI solutions.
About Alicja Kwasniewska, Ph.D.
Alicja is a Senior Principal ML Architect at SiMa.ai with 10 years of experience in the industry, specializing in Artificial Intelligence applications and focused on reshaping field operations and unlocking new levels of efficiency, security, and real-time decision making across various industries. She’s recently received the AI Excellence Award from Perspektywy among other top 10 women in AI. As an author of 30+ high-impact journals and international conference publications in AI, Alicja focuses on advancing knowledge in the multimodal data processing domain with deep learning. She is also a co-chair of the AI in Aviations Use Case group in the Society of Automotive Engineers Organization, helping to advance mobility knowledge and solutions for the benefit of automotive engineering. In her Ph.D. dissertation, she proposed novel AI models aimed at improving the accuracy of remote healthcare solutions. Alicja is also a co-founder and organizer of the International Summer School on Deep Learning (dl-lab.eu), educating future generations on the fundamentals of deep learning methods.
The content & opinions in this article are the author’s and do not necessarily represent the views of RoboticsTomorrow
Comments (0)
This post does not have any comments. Be the first to leave a comment below.
Featured Product
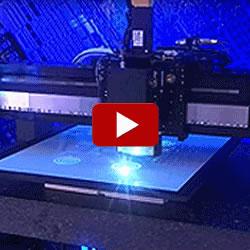